A Novel Measurement-Based Model for Calculating Diffusive Fluxes Across Substrate-Water Interfaces of Marine Aggregates, Sediments and Biofilms
- 1MARUM – Center for Marine Environmental Sciences, University of Bremen, Bremen, Germany
- 2Department of Physics & Earth Sciences, Jacobs University Bremen, Bremen, Germany
- 3Department of Experimental Limnology, Leibniz-Institute of Freshwater Ecology and Inland Fisheries (IGB), Berlin, Germany
- 4Alfred Wegener Institute, Helmholtz Centre for Polar and Marine Research, Bremerhaven, Germany
- 5Department of Geosciences, University of Bremen, Bremen, Germany
- 6HGF-MPG Joint Research Group for Deep Sea Ecology and Technology, Max Planck Institute for Marine Microbiology, Bremen, Germany
- 7Institute for Biochemistry and Biology, Potsdam University, Potsdam, Germany
- 8Department of Marine Sciences, University of Gothenburg, Gothenburg, Sweden
- 9Department of Biogeochemistry, Max Planck Institute for Marine Microbiology, Bremen, Germany
Our understanding of the small-scale processes that drive global biogeochemical cycles and the Earth’s climate is dependent on accurate estimations of interfacial diffusive fluxes to and from biologically-active substrates in aquatic environments. In this study, we present a novel model approach for accurate calculations of diffusive fluxes of dissolved gases, nutrients, and solutes from concentration profiles measured across the substrate-water interfaces using microsensors. The model offers a robust computational scheme for automatized determination of the interface position and enables precise calculations of the interfacial diffusive fluxes simultaneously. In contrast to other methods, the new approach is not restricted to any particular substrate geometry, does not require a priori determination of the interface position for the flux calculation, and, thus, reduces the uncertainties in calculated fluxes arising from partly subjective identification of the interface position. In addition, it is robust when applied to measured profiles containing scattered data points and insensitive to reasonable decreases of the spatial resolution of the data points. The latter feature allows for significantly reducing measurement time which is a crucial factor for in situ experiments.
Introduction
Biogeochemical functions and microbial respiration are key processes for our understanding of the aquatic element cycling (Jørgensen, 2000) and, to a large extent, related to the substrate-water interfaces, e.g., aggregate-water and sediment-water interfaces. Our knowledge on processes of these highly dynamic and heterogenous interfaces, however, is still limited because they occur at microscopic scale of microbial communities (Alldredge and Cohen, 1987; Azam and Long, 2001; Azam and Malfatti, 2007) and also include interfaces with distinct physicochemical properties (Røy et al., 2002; Jackson, 2012; Seymour et al., 2017; Klawonn et al., 2020). Insights into metabolic processes at these interfaces are often achieved by using microsensors to measure solute concentrations in the associated diffusive boundary layer (DBL) within which the molecular diffusion is the dominant solute transport mechanism (Jørgensen and Revsbech, 1985; Gundersen and Jørgensen, 1990; Kühl and Revsbech, 2001; Ploug, 2001). The DBL is formed in a thin water layer surrounding all biologically active marine particles, including individual free-living microorganisms and phytoplankton cells, colonies of microorganisms, aggregates formed from detritus, or fecal pellets (Simon et al., 2002; Iversen et al., 2010, 2017; Belcher et al., 2016). It is also formed above seafloor sediments (Glud, 2008) and around marine biofilms (Lewandowski, 2000; Flemming and Wuertz, 2019). Microsensor measurements are largely non-destructive, measure in real-time, and allow high spatial resolution profiling across interfaces of marine substrates and seawater due to a microsensor tip size of down to 2 μm (Revsbech and Ward, 1983; Revsbech and Jørgensen, 1986; de Beer et al., 1993). Measured O2 concentration profiles have been extensively used to calculate O2 fluxes to and from marine substrates and subsequently to derive metabolic rates of, e.g., respiration and photosynthesis (Jørgensen and Revsbech, 1985; Paerl and Bebout, 1988; Ploug et al., 1997, 1999; Berg et al., 1998, 2003; Ploug and Grossart, 2000; Glud, 2008; Eichner et al., 2017).
Several methods have been developed for calculations of benthic interfacial diffusive fluxes from measured concentration profiles in the sediment or in the overlying water of sediment (Jørgensen and Revsbech, 1985; Reichert, 1994; Urban et al., 1997; Berg et al., 1998; Hondzo et al., 2005; Glud, 2008; O’Connor and Harvey, 2008). Common for these methods is that interfacial fluxes can only be accurately calculated if the precise position of the sediment-water interface has been determined simultaneously. Yet, the accurate determination of the interface from a solute profile is challenging and often manual interpretation is necessary. This became apparent when Bryant et al. (2010) compared five different methods for the benthic O2 flux calculation and obtained almost similar results when comparing estimated fluxes and DBL thicknesses. However, the authors argued that one factor for the deviations in the results is the definition of the sediment-water interface in different methods. For marine particles (e.g., aggregates and phytoplankton colonies), it is particularly important to precisely determine the surface interface as the particle shape and size may impact the exchange of solutes between particles and surrounding water (Zetsche et al., 2020). Assuming spherical particle geometry as well as constant consumption rate inside marine particles, Ploug et al. (1997) developed a model for flux calculation into (and out of) marine particles. In that model, the analytical solutions of the reaction-diffusion equation inside and the diffusion equation within the DBL around the particle were fitted to the measured solute profile and the associated model parameters were optimized to yield mass conservation—total consumption rate inside the particle must be equal to the corresponding area-integrated interfacial diffusive flux at steady state.
To improve the precision of calculating interfacial diffusive fluxes from measured concentration profiles, we here present a general (and computationally efficient) model for accurate and simultaneous determinations of both the substrate-water interface position and the interfacial flux. The method differs from previous methods, in that (i) it doesn’t require a prior determination of the substrate-water interface position for flux calculations, (ii) it is based on the full mass transfer equation (advection-diffusion-reaction equation), and (iii) it is not restricted to any particular surface geometry of the substrates. Thus, both the interface position and flux are calculated objectively. In addition, it is robust when the measured concentration data points fluctuate in space and time—which is often the case when measuring in situ—or in situations where the spatial resolution of data points has to be decreased due to constrains in measurement time.
In the following, we present our new model approach and validate it using a number of previously published O2 profiles measured in marine aggregates and seafloor sediments. The obtained results are compared with those of previous methods and the advantages and extent of applications of the approach are discussed. Henceforth, for ease of referring, we name our approach as “Derivative-Max” model and refer to the model of Ploug et al. (1997) as “Sphere” model.
Materials and Equipments
Microsensor Profiling
Marine Aggregates
O2 profiles measured across the aggregate-water interface considered in this study (Figures 1, 2) have already been used to calculate O2 specific respiration rates in cyanobacterial aggregates by Klawonn et al. (2015) and sinking aggregates by Stief et al. (2016) and Iversen and Ploug (2010). Aggregates were sampled in the field (Klawonn et al., 2015) or formed from Skeletonema marinoi (Stief et al., 2016) or E. huxleyi and S. costatum (Iversen and Ploug, 2010) in roller tanks (Shanks and Edmondson, 1989). Oxygen profiles were measured in a net-jet flow system (Ploug and Jørgensen, 1999) using a Clark-type oxygen microelectrode with a guard cathode (Revsbech, 1989) mounted in a micromanipulator and calibrated at air-saturation and at anoxic conditions. The electrode current was measured on a picoamperemeter (Unisense, PA2000) and read on a strip chart recorder (Kipp and Zonen) at high resolution (2 μmol O2 cm–1). The tip diameter of the microsensor was 2–10 μm.
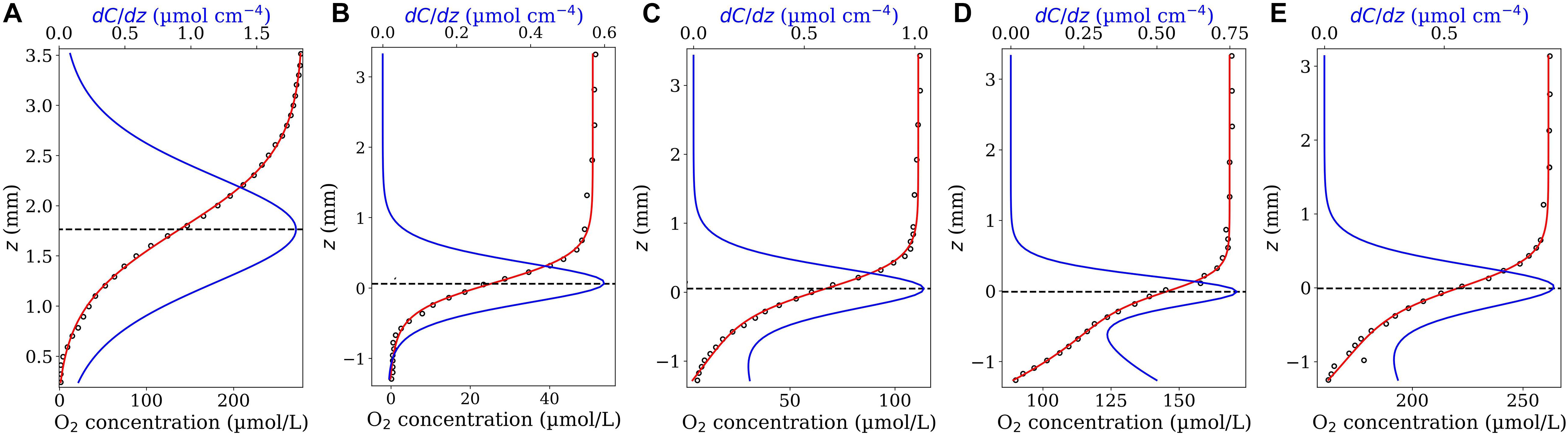
Figure 1. Model validation. O2 concentration profiles (circle symbols) measured across the aggregate’s surface (from the ambient water down towards the aggregate’s center) within a representative N. spumigena aggregate (A) and a representative Skeletonema marioni aggregate exposed to different air saturation levels: 15% (B), 40% (C), 75% (D) and 100% (E). The data shown in (A) and (B–E) were digitalized from Figure 2 in Klawonn et al. (2015) and Figure 1 in Stief et al. (2016), respectively. The z values obtained from Figure 1 in Stief et al. (2016) were multiplied by −1 setting the positive direction of z-axis upwards. The red curves show the fitted model functions [Eq. 4 in (A) and Eq. 7 in (B–E)] and the blue curves the corresponding first derivative functions. The horizontal dashed lines indicate the position of the interface on the z-axis, i.e., the position corresponded to the maximum value of the first derivative function.
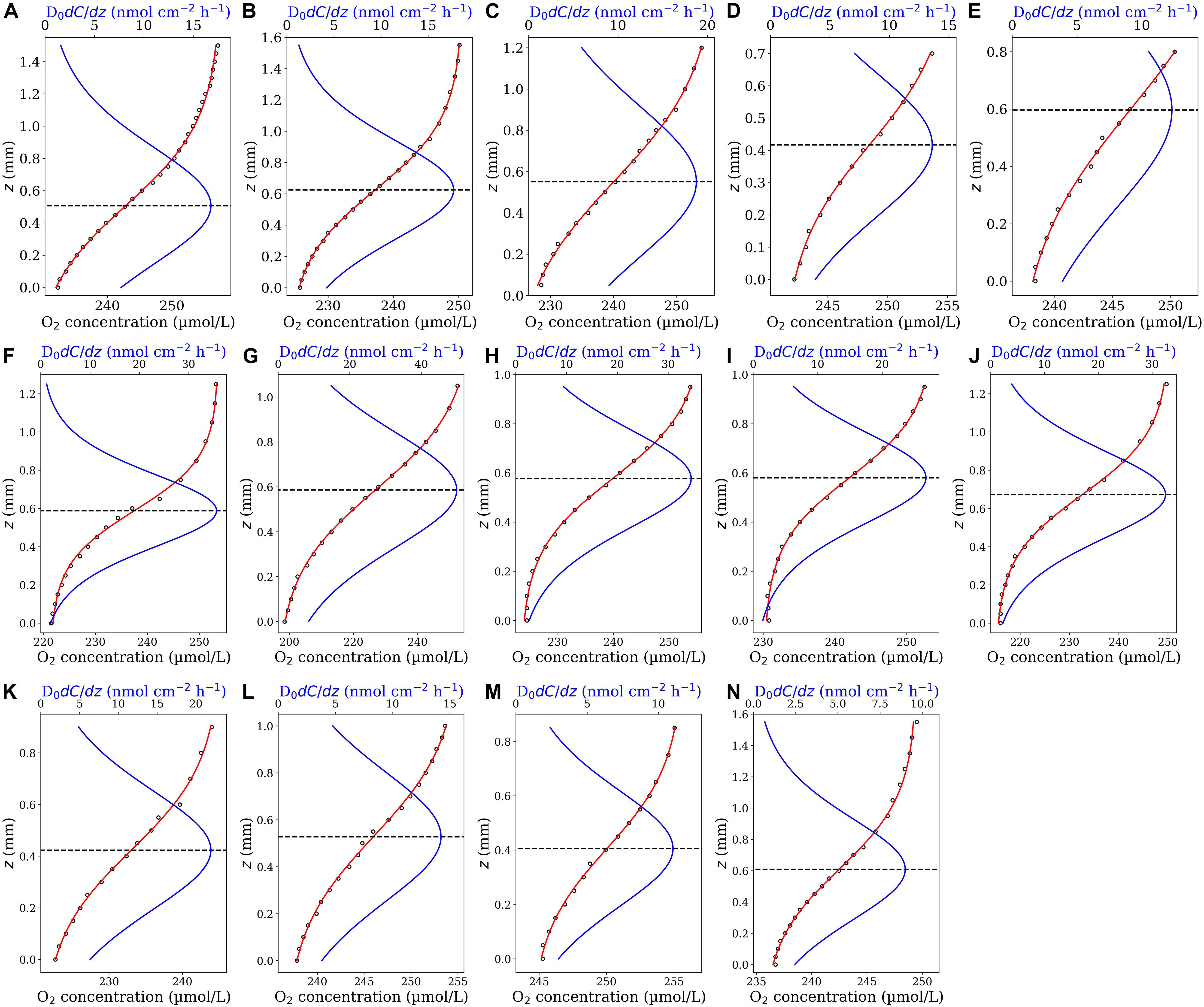
Figure 2. O2 concentrations profiles (close circles) measured in sinking diatom aggregates (A–E), coccolithophore aggregates (F–J) and mixture of diatom and coccolithophore aggregates (J–N) (Iversen and Ploug, 2010). The highest and lowest concentrations represent the onset of concentration variation in the ambient water above the aggregate (ambient concentration) and the concentration in the middle of each aggregate (minimum concentration), respectively. The origin of the z-axis (z = 0) has been set at the middle of the aggregates with the positive direction upwards. The red curves are the best fit of the first fit-model function (Eq. 4). The blue curves represent the corresponding diffusive flux functions (Eq. 5 multiplied by the diffusion coefficient). The horizontal dashed lines identify the position of aggregate-water interface (corresponding to the maximum diffusive fluxes).
Seafloor Sediment
O2 profiles measured across the sediment-water interface considered in this study (Figure 3) were digitalized from Figure 2 in Jørgensen and Revsbech (1985). The sediment was collected in the Aarhus Bay (Denmark) during summer 1982. The O2 concentration measurements were performed using microelectrodes with a gold-coated tip of 5–10 μm, made according to Baumgärtl and Lübbers (1973, 1983) and Revsbech (1983). The measurement was repeated 10 times in the same sample. The sediment-water interface was determined using the electrode vibration method and set to z = 0.0 [see Jørgensen and Revsbech (1985) for details].
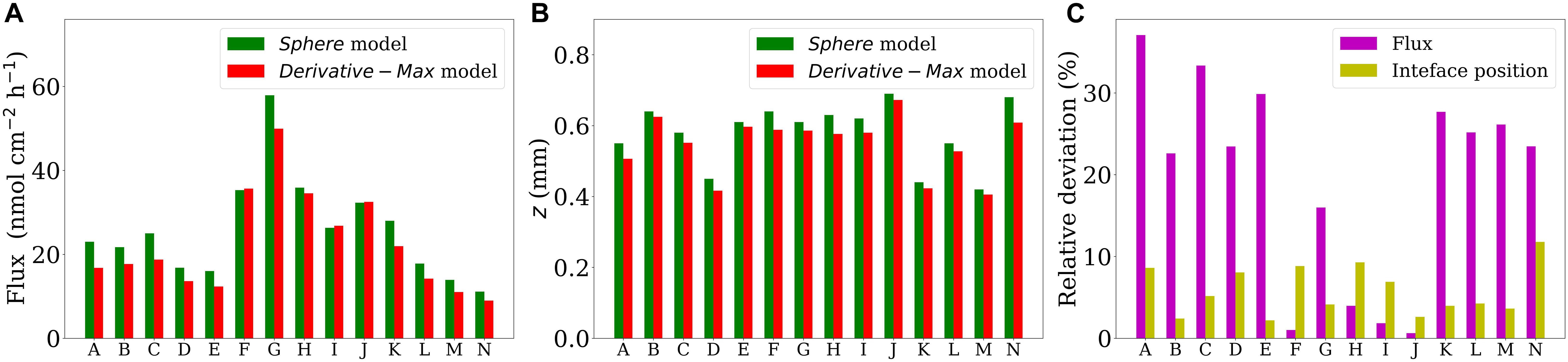
Figure 3. Head-to-head comparison of the fluxes (A) and the interface positions (B) obtained by using the Sphere and Derivative-Max models applied on all the 14 profiles shown in Figure 2. Panel (C) shows the corresponding relative deviations with respect to the results of Derivative-Max model for each profile.
In this work, digitalization of all data from previously published concentration profiles was carefully performed using the WebPlotDigitizer software1. The associated errors in the obtained positions of the data on the z (depth) and x (concentration) axes were less than 1% and were therefore insignificant.
Methods
Model Description
Derivative-Max Model
Transfer of solutes and dissolved gases to and from sinking aggregates, phytoplankton colonies and sediments can be formulated as a 3-dimensional advection-diffusion-reaction equation (Nield and Bejan, 1998; Moradi et al., 2018):
where the parameters ε, C, t, u, D, and R represent substrate porosity, solute concentration, time, fluid velocity (flow), diffusion coefficient and biological reaction term, respectively. In the above equation, the symbol “.” denotes the dot product and “∇” the gradient operator. The latter is defined as where and represent the unit vectors along x, y, and z directions, receptively. Technically, due to the often unknown form of reaction term and complex surface topography, it is very difficult to analytically solve Eq. 1 for a real system. However, Eq. 1 can be simplified at the substrate-water interface with the help of some justified assumptions. In situations in which the flow becomes insignificant at substrate-water interface we can assume the no slip boundary condition, i.e., u = 0 at the substrate surface. At the immediate vicinity of the substrate surface in the water domain, the porosity is equal to unity (ε = 1), the microbial reaction term is assumed to be zero (R = 0) as compared to that of in the substrate, and the diffusion coefficient is considered to be constant (often equal to its value in the ambient water, D0). Therefore, the steady-state mass transfer equation at the substrate-water interface is reduced to:
This equation implies that the “second derivative” of the concentration function, C(x,y,z), becomes zero at the interface. If the concentration function is known, Eq. 2 can thus be used as a criterion for determining the interface position. Note that this condition is locally valid and independent of the surface topography and type of microbial reactions. Once the position of the interface is determined, the interfacial diffusive flux is calculated using Fick’s 1st law as −D0∇C(x,y,z).
The concentration measurements using microsensor (profiling) are often performed along the z-direction perpendicular to the substrate surface crossing the interface. Thus, the local concentration variation along x and y directions can be often neglected (at the interface) and the interface criterion (Eq. 2) can be applied along the z-direction:
If a measured concentration profile is characterized by a representative mathematical function, C(z), Eq. 3 can be then directly applied to determine the accurate position of the interface, zi. Mathematically, the interface criterion (Eq. 3) is met at z positions in which the first derivative function of C(z), i.e., dC(z)/dz, has a local maximum or minimum (hill or valley). This is because the first derivative (slope) of a mathematical function f(z) (here f(z) = dC(z)/dz) is zero wherever the function has a local maximum or minimum. Since the diffusive flux cannot be a minimum at the interface, it is expected that the absolute values of dC(z)/dz, i.e., |dC(z)/dz|, shows a local maximum and this determines the position of the interface. The reason for using the absolute values is to account for both negative (solute consumption) and positive (solute production) fluxes. At the same time, the maximum of |dC(z)/dz| calculates the diffusive flux when multiplied by the diffusion coefficient.
In the following, we introduce two appropriate mathematical functions that could accurately fit measured solute concentration profiles across the substrate-water interface of marine aggregates and sediments.
Standard Hyperbolic Tangent Fit-Model Function
The examination of several oxygen profiles (measured along the z-axis) in sinking aggregates and phytoplankton colonies demonstrated that O2 concentration profiles across the interface typically follow a mathematical function of the form:
where the tanh function is defined as tanh(x) = (e(x)−e(−x))/(e(x) + e(−x)) and α, β, γ, and δ are free parameters to be determined by the best fit of Eq. 4 on the considered profile. The corresponding first derivative of C(z) can be formulated as:
Since tanh2((z + γ)/δ)is ≥ 0, the maximum value of dC(z)/dz amounts to β/δ which is obtained at z = –γ. This means the interface criterion, Eq. 3, is met at z = –γ, while γ determines the location of the interface on the z-axis. Accordingly, the corresponding interfacial diffusive flux amounts to Ji = D0β/δ. Note that Eq. 4 has been constructed in a way that the obtained fitting parameters can provide all the information needed without the need to further survey the first derivative equation (Eq. 5). This information includes the position of the interface as well as the corresponding interfacial diffusive flux. For methodological reasons and visual purposes, however, we plotted both the fitted Eq. 4 and the corresponding first derivative, Eq. 5, for all the examined profiles in this study.
Distorted Hyperbolic Tangent Fit-Model Function
A distorted tanh function can be applied in situations where the mathematical shape of measured profiles deviates from the standard tanh function (Eq. 4). Such profiles are often measured across interfaces of substrates with low biological activities particularly in sediments or in situations where the flow has strong effect on the profiles. An appropriate distorted tanh function can be constructed as:
in which, as compared to Eq. 4, two additional fitting parameters, μ and σ, have been added. Accordingly, the extended version of Eq. 4 reads as:
Since Eq. 7 has two additional fitting parameters, it is more flexible to fit the measured concentration profiles. Its first derivative, i.e., dCext.(z)/dz, on the other hand, has a rather complex form (see Eqs. 8–13) and its maximum, in contrast to Eq. 5, cannot be given in terms of a simple relation of fitting parameters. However, once the fitting parameters are determined, one can easily evaluate and plot dCext.(z)/dz for the considered range of z positions and determine its maximum value, and thus the interface position, zi. This can be readily done with the available built-in functions in all scripting languages such as Python and R, or in Excel at almost no computational cost (in a fraction of a minute). The maximum of |dCext.(z)/dz| multiplied by the diffusion coefficient gives the diffusive flux at the interface. The first derivative of Cext.(z) can be formulated as:
in which
Computational Procedure
(i) The measured profile across the interface is fitted with an appropriate mathematical function, C(z), i.e., Eq. 4 or 7, and the fitting parameters are determined. The considered profile (or profile portion) must include data points measured in both sides of the substrate surface, i.e., the substrate and the overlaying water of substrate. For the marine particles, a portion of the profile down to the particle center where the minimum concentration—or maximum in the case of solute production— has been measured can be selected. For calculations, the positive direction of z-axis is considered to be upwards (the position value of microsensor on the z-axis decreases as it goes down toward the substrate).
(ii) The first derivative of the fitted function, i.e., dC(z)/dz, is analytically calculated and numerically evaluated and plotted for the considered range of z positions.
(iii) The position on the z-axis at which dC(z)/dz shows a local maximum determines the interface position zi.
(iv) The product of the diffusion coefficient, D0, and the maximum value of the first derivative function gives the interfacial diffusive flux, i.e., Ji = D0 × max [dC(z)/dz]. Since it was assumed that the interface is situated at the immediate vicinity of the substrate surface in the water domain, the diffusion coefficient of the solute in the ambient water has been used.
As mentioned already, the sign of dC(z)/dz can be positive or negative at the interface, depending on the type of biological reaction (consumption or production). Therefore, the maximum value of |dC(z)/dz| is used to determine the position of the interface and the diffusive flux.
Model Validation
Figure 1A shows the best fit of the first fit-model function, Eq. 4, on an O2 profile measured in a N. spumigena aggregate together with the corresponding first derivative function, Eq. 5. The suggested fit-model function represented well the measured profile [data were taken from Figure 2 in Klawonn et al. (2015)]. The maximum value of dC(z)/dz (= 1.799 μmol cm–4) occurred at zi = 1.763 mm very close to the localized aggregate surface reported in the original study (z = 1.83 mm). Using the reported diffusion coefficient (D0 = 1.96 × 10–5cm2s–1) the interfacial diffusive flux equated to Ji = 126.94 nmol cm–2 h–1. This agrees well with the reported flux of 126 nmol cm–2 h–1 calculated by Klawonn et al. (2015).
Figures 1B–E show the best fits of the second fit-model function, Eq. 7, on the O2 profiles measured across a Skeletonema diatom aggregate (exposed to different O2 air-saturation levels) together with the corresponding first derivative function (Eq. 8). The measured profiles were digitized from Figure 1 in Stief et al. (2016). The aggregate surface was localized at z = 0.0 in the original study. As shown, the second fit-model function can adequately represent all the profiles and the maxima of the corresponding first derivative function could accurately reproduce the reported interface position, i.e., they occur at z positions very close to z = 0.0 in all the cases. Accordingly, the interfacial diffusive fluxes equated to Ji = 36.02, 62.58, 46.55, 57.51 nmol cm–2h–1, respectively, at 15, 40, 75, and 100% air saturation levels (D0 = 1.67 × 10–5cm2s–1). The associated diffusive fluxes have not been explicitly reported for these profiles in the original study which hinders a direct flux comparison.
Additional validations for the model outputs can be also perceived in the “Results” section where further comparisons have been presented.
Results
We applied the Derivative-Max model to calculate the flux and interface position for 14 oxygen concentration profiles measured through different types of sinking aggregates (see section “Microsensor Profiling”). Eq. 4 accurately represented the measured O2 profiles in all tested cases (Figure 2, red curves). Combing the steps (iii) and (iv) explained in the section “Computational Procedure”, the corresponding diffusive flux function, i.e., Eq. 5 multiplied by the diffusion coefficient (D0 = 1.714 × 10–5cm2s–1), has been plotted for all the profiles (Figure 2, blue curves). The maximum (pick) of the flux function retuned the interfacial diffusive flux, and its position on the z-axis simultaneously determined the optimum location of the aggregate-water interface (displayed by horizontal dashed lines). Interestingly, as seen, the flux function shows a local maximum in all the tested cases. It should be noted that, as mentioned before, if Eq. 4 is used to fit the concentration profiles, one only needs to determine the fitting parameters to calculate the interfacial diffusive flux and the interface location, i.e., Ji = −D0β/δ and zi = −γ, respectively. For methodological reasons, we plotted the fitted as well as the corresponding diffusive flux functions for the considered range of z positions in all the cases. We applied the Sphere model (Ploug et al., 1997) on these profiles to provide direct comparisons of calculated fluxes and the interface positions using both the models (Figure 3). The positions of the interfaces obtained by applying the Sphere model were slightly larger than those by Derivative-Max model, and the Sphere model overestimated ca. 21% (in average) diffusive fluxes compared to the Derivative-Max model. To understand the potential source of this flux difference, the best fitted functions obtained from both the models have been compared for each profile (Figure 4). Since both models used the least square method to find the best fit of profiles the residual sum of squares (RSS) is also shown for each profile in the inset plots (Figure 4). As seen quantitatively from the RSS plots (and also visually) the Derivative-Max model significantly improved the quality of the fitted functions. That is because the Derivative-Max model does not need assumptions on the geometry of the substrate or the type of reaction inside the particles while the Sphere model assumes spherical geometry as well as constant volumetric consumption rate inside the aggregates (Ploug et al., 1997). In addition, the Derivative-Max model does also not exclude the effect of advection on the measured profiles in the DBL. Note that all the aggregate-water interface profiles considered here have been measured in aggregates subject to flow.
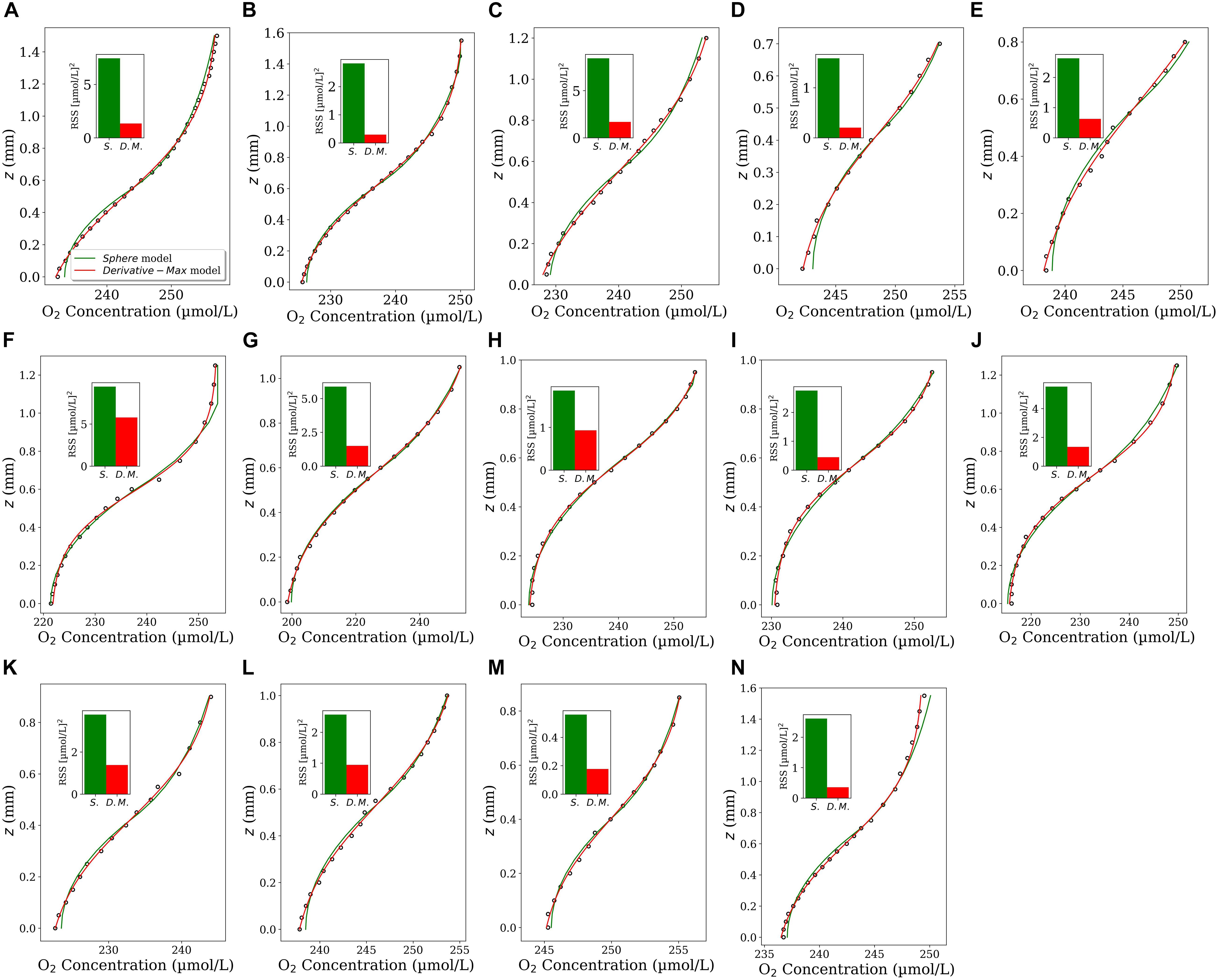
Figure 4. Comparison of the best fit-model functions obtained by using the Sphere (green color) and the Derivative-Max (red color) models applied on all the 14 profiles (A–N) shown in Figure 2. The inset plots show the residual sum of squares (RSS) for the best fit of each model.
We applied the second fit-model function, Eq. 7, to fit O2 profiles measured in seafloor sediments (see section “Microsensor Profiling”). While Eq. 4 could not accurately fit these profiles, Eq. 7 could adequately represent all the measured profiles (Figure 5, red curves). All curves of the corresponding diffusive flux functions (Eq. 8 multiplied by the reported diffusion coefficient D0 = 2.0 × 10–5cm2s–1) showed a local maximum, which determined the interfacial flux and the location of the interface on the z-axis. Interestingly, in all cases, the maximum of the diffusive flux occurred around z = 0. This is the position that has been identified as the interface position in the original study [see Figure 2 in Jørgensen and Revsbech (1985)]. The fluxes and interface positions obtained from the Derivative-Max model are given in Table 1. The average flux amounts to 0.129 ± 0.0149 μmol cm–2 h–1 (n = 10) which agrees with the reported value of 0.111 ± 0.004 μmol cm–2 h–1 in the original study. Since these measurements are replicate measurements of the same sediment it is also interesting to make a single fit on all data points of the 10 profiles. The fitted function (Eq. 7) on the pooled data and the corresponding diffusive flux function are shown in Figure 6A. This way, the flux amounted to 0.125 μmol cm–2 h–1 with the interface to be at z = −0.083 mm which again are in line with the reported values. Note that, in the plots presented here we simply applied D0 also for the sediment domain (below the horizontal dashed lines). Yet, the diffusive flux of the solute below the interface in the sediment domain has to be calculated as J = −φDsdC(z)/dz in which Ds is the diffusion coefficient of the considered solute in the sediment (Glud, 2008; Bryant et al., 2010). This, however, has no effect on the flux calculation in our model since the interface criterion (Eq. 3) was derived under the assumption that the interface is situated in the immediate vicinity of the substrate surface in the water domain. In fact, we are solely interested in the maximum value of the diffusive flux curve—that occurs at the interface—and not the rest of the curve.
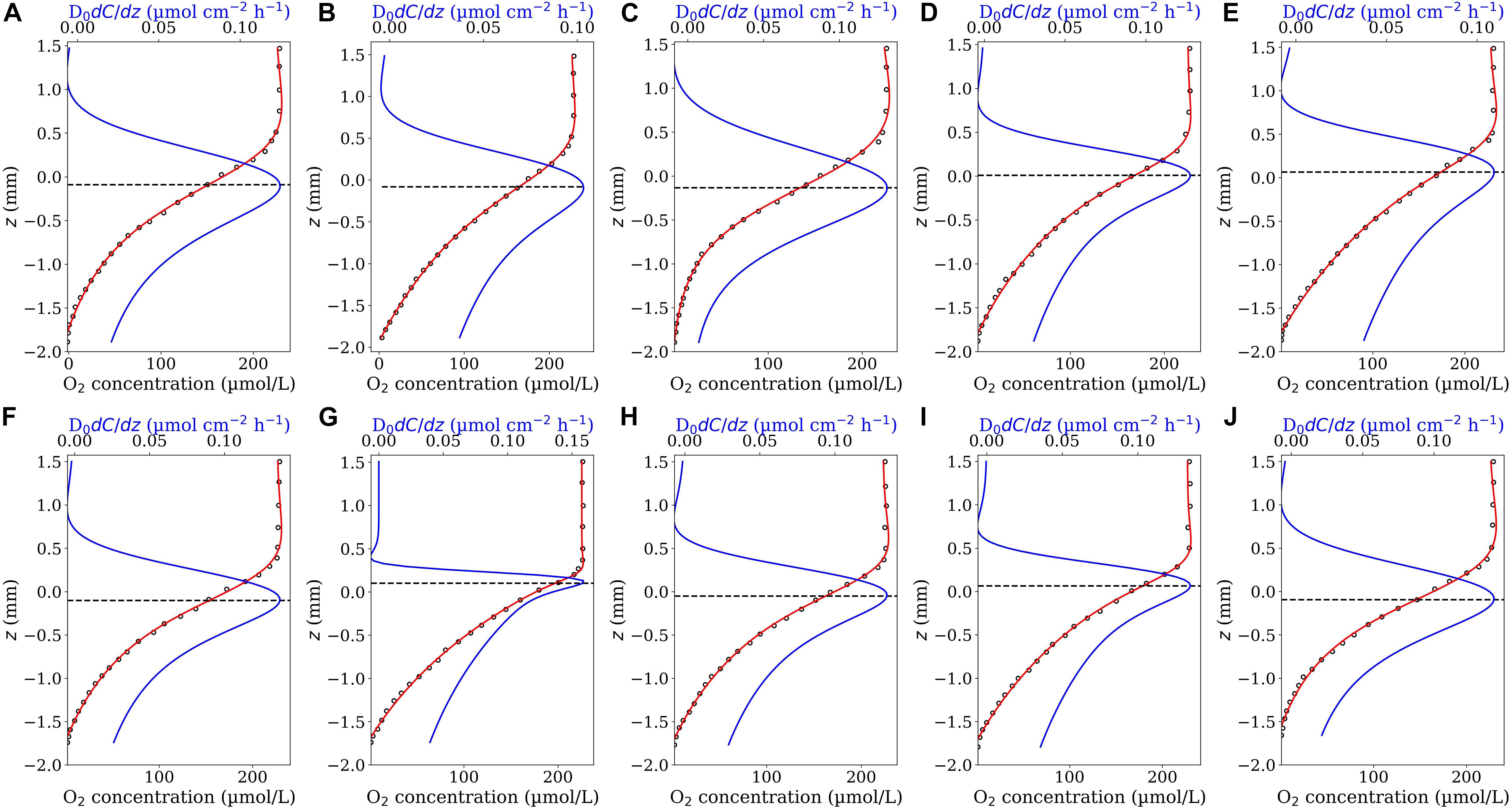
Figure 5. Ten O2 concentrations profiles (A–J) measured in the sediment as a function of the z position (close circles). The data were digitalized from Figure 2 in Jørgensen and Revsbech (1985). The red curves are the best fit of Eq. 7 on the profiles. The blue curves represent the corresponding diffusive flux functions (Eq. 8 multiplied by the diffusion coefficient). The horizontal dashed lines indicate the position of the sediment-water interface (corresponding to the maximum diffusive fluxes).

Table 1. Fluxes and interface positions in the considered sediment (Figure 5) obtained by using the Derivative-Max model.
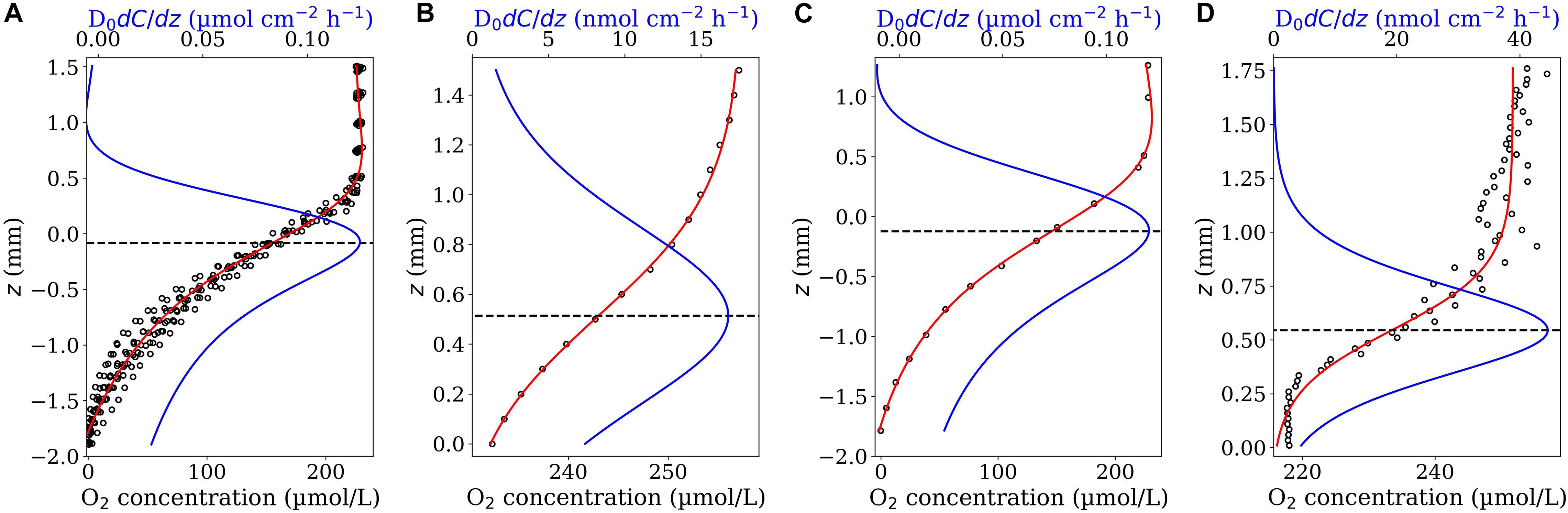
Figure 6. Outputs of Derivative-Max model applied on different type of measured profiles: Replicated profiles (A), low resolution profiles (B,C), and noisy profiles (D). The profiles in panel (A) are pooled data of all the 10 profiles shown in Figure 5. Panels (B,C) show the profiles considered in Figures 2A, 5A in which every second data point has been removed, resulting a coarser profile of 100 μm resolution for the considered aggregate (B) and ca. 200 μm for the sediment (C). Panel (D) shows an example noisy profile containing scattered data points. Eq. 7 and 4 (red curves) have been used to fit the profiles shown in (A,C) and (B,D), respectively. Blue curves show the corresponding diffusive flux functions and the dashed lines determine the position of interface on the z-axis (corresponding to the maximum diffusive fluxes).
To test the robustness of the Derivative-Max model we considered two different cases. The first case included O2 profiles with lower spatial resolutions. We reduced the spatial resolution of the profiles shown in Figure 2A and Figure 5A by a factor of 2 and applied the model on the resulted (coarser) profiles (Figures 6B,C). As seen, in both cases, the calculated flux and interface position are very close to those obtained from the original profiles. The second case included noisy profiles containing scattered data points. Figure 6D shows an example of a noisy O2 profile measured in an aggregate subject to irregular (nonlaminar) flow. As shown, the Derivative-Max model could calculate reasonable interface position and diffusive flux from the noisy profile.
Discussion
Calculating Interfacial Fluxes
Sinking marine aggregates and phytoplankton colonies are essential for the sequestration of CO2 from the atmosphere in the ocean’s interior and sediments. Therefore, accurate quantification of associated microbial processes and small-scale mass transfers is crucial for understanding large-scale biogeochemical processes in the ocean (Azam and Malfatti, 2007; Seymour et al., 2017). Precise determination of the position of the substrate-water interfaces is pivotal for accurate calculation of the interfacial fluxes. In addition, localization of the interface position is also important for estimating the volume or surface area of marine particles if biological volumetric rates are of interest (e.g., Iversen and Ploug, 2010). However, the surface of marine substrates is often rugged at the microscale level and thus localization of the interface can be arguable and might be biased by the executer’s interpretation. In previous studies, the interface position has been often localized either by microscopy during data acquisition (e.g., Ploug and Jørgensen, 1999), via the interpretation of the slope of the measured profiles, assessment of the O2 concentration standard deviations or visual interpretation of the profile itself (Wenzhöfer et al., 2001; Røy et al., 2004; Brand et al., 2007; Bryant et al., 2010; Klawonn et al., 2015). To some extent, all these approaches require subjective decisions. For instance, Figure 7 demonstrates how the angle of a camera lens or a microscope may bias the precise localization of the true substrate surface or the slope of a profile can be calculated differently based on the choice of data considered. In our model, the suggested interface criterion, d2C(z)/dz2 = 0, has been derived from the mass transfer equation. Therefore, the determination of the interface is made objectively which leads to fewer biases in both determination of interface position and flux calculation. Indeed, the Derivative-Max model reproduces same results when analyses are repeated by another model executer for the same O2 profile.
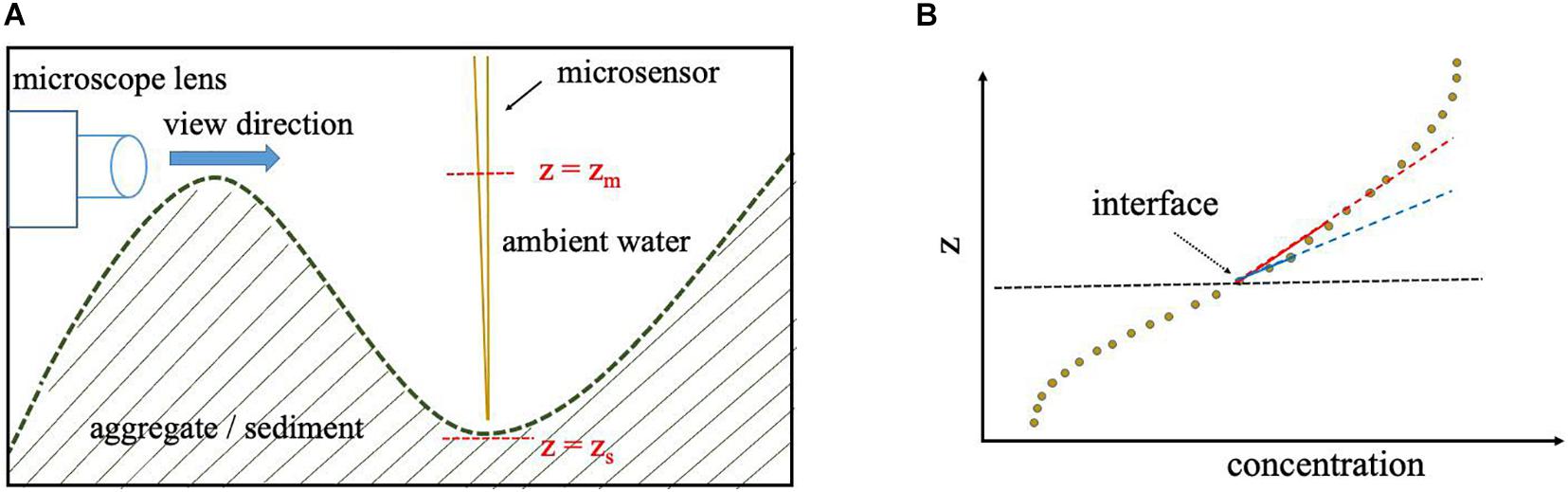
Figure 7. While the interface is at z = zs, the interface might be mistakenly determined by microscopy to be located at z = zm, due to the surface roughness as shown by a simplified 2D sketch (A). If the concentration data points used for linear fitting are not adequately close to the interface the concentration gradient (slope) might be underestimated as illustrated by the fitted blue and red lines (B).
Interface Criterion
The suggested interface criterion theoretically implies that the concentration profile varies linearly “exactly” at the interface, i.e., a linear solution, C(z) = Az + B (A and B are two constants) meets the criterion at the interface. This local linear concentration variation is then interpolated by a proper representative function (Eq. 4 or 7), and its position is exactly and easily specified (where the first derivative of the fitted function is maximal). Notably, together with the interface position, the corresponding interfacial diffusive flux is calculated simultaneously. Assuming flat geometry for the substrate surface as well as negligible flow effects in the substrate’s overlaying water—which is often the case in deep-sea sediments—the mass transfer equation can be practically reduced to a one-dimensional problem along the z-axis and the interface criterion will be also met in the overlaying water. Therefore, the linear concentration variation is extended through the DBL and the concentration gradient can be directly obtained from the slope of the linear portion of the measured profile in the DBL, the so-called direct method (Jørgensen and Revsbech, 1985; Bryant et al., 2010). In contrast, if the flow effect cannot be neglected and/or the substrate surface cannot be treated as flat, the solute concentration varies nonlinearly in the overlaying water and the flux cannot be accurately determined by a linear approach (Ploug et al., 1997, 2002; Kiørboe et al., 2001; Bryant et al., 2010; Moradi et al., 2018). This is the case in sinking marine particles (aggregates and colonies) or in coarser sandy sediments in which the flow effect in the DBL cannot be neglected.
It should be mentioned that, the interface criterion (Eq. 3) was derived under the assumption of zero flow velocity at the substrate surface. This condition will be met if the substrate is impermeable to flow (e.g., in sinking marine aggregates) or where the flow effect at the interface is negligible (e.g., in many deep-ocean sediments). In situations in which a permeable substrate, such as a sandy sediment, is subject to a strong advective flow which is often the case in the shelf waters or in rivers, two scenarios can be considered. First, the water flow is parallel to the substrate surface and has a negligible vertical component along the z-axis through the substrate. In this case, presuming that the local concentration variations along the x and y axes are insignificant at the interface—that is a reasonable assumption if the profiling is performed normal to the substrate surface—the term u(x,y,z).∇C(x,y,z) in Eq. 1 can be neglected and, thus, Eq. 3 can still serve as a criterion at the interface, providing reliable estimates of the interface position. Second, if the water velocity has a nonnegligible vertical component through the substrate, uz, the interface criterion becomes a more complex equation including the velocity components, and the diffusive flux may not be maximal at the interface anymore. In these situations, the fluid velocity at the substrate surface is often unknown and, thus, solving such equation to determine the precise position of the interface is not possible. Here, one can still make use of the suggested fit-model functions (Eq. 4 and 7) to fit the concentration profile and calculate their derivatives (gradients) for flux calculation by applying the Fick’s 1st law. However, the position of the interface has to be identified using the previous approaches. Note that, the second fit-model function (Eq. 7) is a robust function that can properly fit a broad range of microsensor profiles and its derivative can be easily evaluated (Eq. 8) at any point at which the interface is assumed to be. Nevertheless, as mentioned before, one should keep in mind that if the gradient of the fitted profile in the substrate domain is considered for flux calculation, the diffusive flux has to be calculated as J = −φDsdC(z)/dz. At the interface, this should be equal to the flux obtained from the gradient of the profile in the water domain, J = −D0dC(z)/dz (Glud, 2008; Bryant et al., 2010).
Advantages
An interesting feature of the present model is that it does not make any strict assumptions neither on the geometry of the substrate nor on the flow effect within the DBL. In addition, the suggested fit-model functions (Eq. 4 and 7) are robust enough to capture linear or nonlinear concentration variation across the interface in both the water and the substrate domains. This increases the field of application of the model and makes it useful to serve as a general approach. Another important feature of the model, as compared to other methods, is that it fits a single function (Eq. 4 or 7) on a large portion of (or entire) the concentration profile measured across the interface (overall fitting). This reduces uncertainty and improves the reproducibility of the calculated fluxes as compared to other existing methods in which (i) a subset of data points is used for finding the slope of the profile at the interface (local fitting) and thus the obtained fluxes may vary depending on the choice of data points; (ii) heterogeneous topography and microbe distribution, irregular flow or unstable experimental conditions cause that the measured concentrations data points get scattered (e.g., see Figure 6D); (iii) the spatial resolution of measured data points is decreased (Figures 6B,C). The latter is important for in situ oxygen measurement in deep-sea sediments measured using autonomous lander systems. Such landers are often equipped with several oxygen electrodes (Reimers, 1987; Glud et al., 1994; Wenzhöfer and Glud, 2002) and can measure numerous profiles simultaneously. Typical profiles cover a depth range of 5–15 cm and require between 1 and 2 h measuring time. However, to save station time at sites with deep oxygen penetration, the overall measurement time is kept short by measuring at a relatively coarse vertical resolution with increment step sizes up to 500 μm (e.g., Wenzhöfer et al., 2016). This rather low spatial resolution often makes it difficult to identify the location of the sediment-water interface from the shape of the profile. The overall fitting approach used in the present model can significantly improve the accuracy of the interface location and we obtained similar results for interface location and diffusive flux when using either a low or high spatial resolution of data points for the same vertical O2 profiles. Due to its efficient performance on standard CPUs, the present model has the potential to be implemented into fully autonomous measuring platforms for long-term investigations or network systems. In this way, the present model can help to set the in situ autonomous measuring frequency in relation to the sedimentation rate of organic matter and associated benthic flux. Thereby, changes in the calculated benthic consumption rate could be used to identify periods of intensified activities at the seafloor and thus trigger other instruments or platforms to cover a larger spatial and temporal resolution. For instance, when using the present model on autonomous mobile platforms routinely measuring once a week [e.g., benthic crawler (Wenzhöfer et al., 2016; Lemburg et al., 2018)] the calculated flux information could be used as a measure of the benthic microbial metabolic rates in the sediment and frequency, depth, and areal coverage of the measured profiles adjusted accordingly– e.g., higher measuring frequency or larger spatial coverage in periods of elevated fluxes.
It is also worth mentioning that the application of the model is not restricted to O2 microsensor profiles. Indeed, the “Derivative-Max” model can also be applied to microprofiles of other solutes such as pH, redox, N2O, etc. (see examples for ammonium and nitrate in Figure 8) provided that the associated diffusive flux is expected to be maximal around the interface. Here, if needed, one may have to further modify the Eq. 4 or 7 to be able to properly fit the considered profiles that belong to a different profile-shape class.
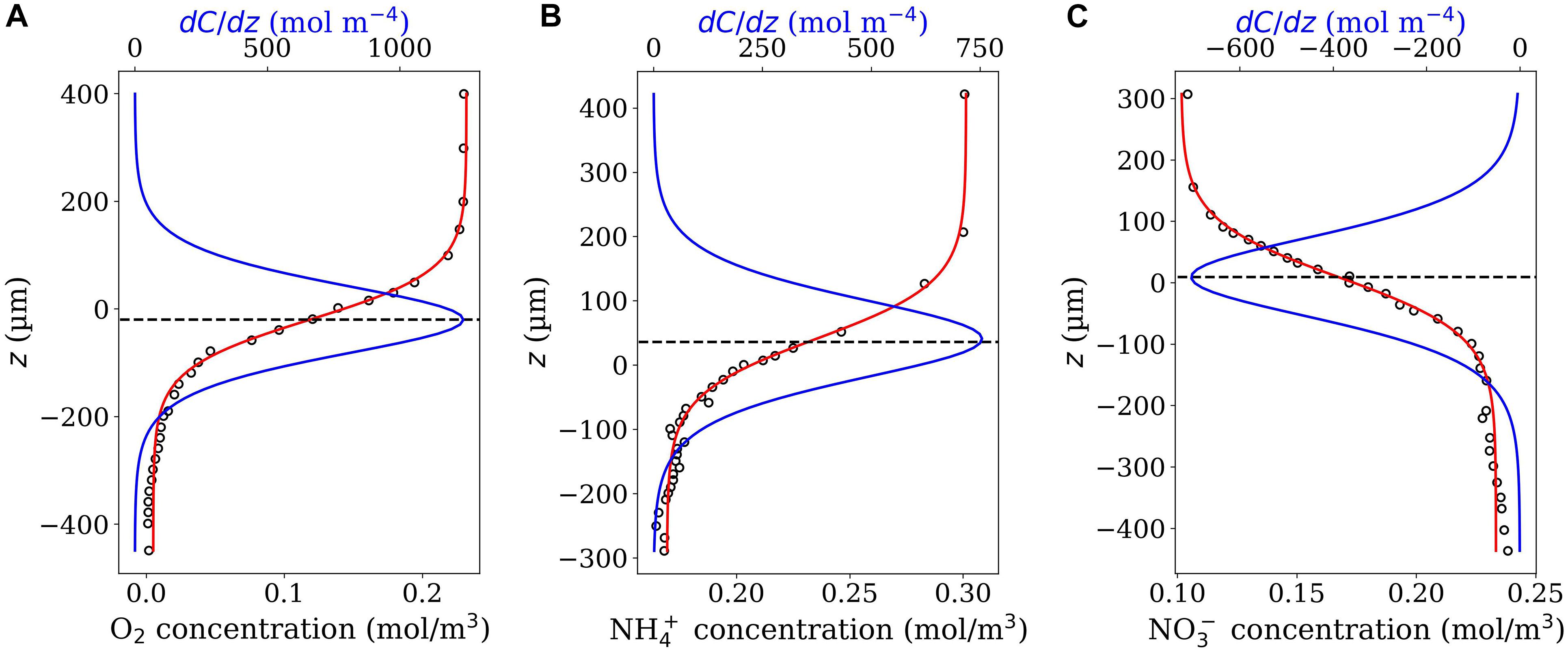
Figure 8. Oxygen (A), ammonium (B) and nitrate (C) microprofiles (close circles) measured in nitrifying bacterial aggregates. Data were digitalized from Figure 3 in de Beer et al. (1993). The obtained z values were multiplied by –1 setting the positive direction of z-axis upwards. As seen, Eq. 4 properly fits all the three profiles (red curves) and the picks of the corresponding derivative function, Eq. 5 (blue curves) occur around z = 0, the position at which the interface has been identified in the original study. Note that, in contrast to the cases of oxygen and ammonium, the nitrate concentration increases gradually going from the bulk water towards the aggregate center, and that is why the values of the derivative function is negative for the nitrate microprofile.
Conclusion
The Derivative-Max model offers a robust computational scheme for automatized and simultaneous determination of the interface position and calculation of diffusive flux of measured concentration profiles. Calculations are independent of any other subjective parameters (e.g., visible inspection of the substrate surface) and thus results obtained more objectively. This is of particular interest when full automatized systems are used, e.g., on autonomous robotic deep-sea platforms.
Data Availability Statement
The script of the computer program for the model and all the datasets used in the article can be directly requested from NM, nmoradi@marum.de.
Author Contributions
NM developed the new model, provided the model outputs, and with substantial inputs from all co-authors wrote the manuscript. NM, IK, MI, H-PG, and AK designed the study. MI and HP provided the oxygen profiles measured in marine aggregates presented in the “Results” section and the associated results obtained by using the “Sphere” model. All authors critically discussed the results, significantly contributed in the “Discussion” section and revised the manuscript.
Funding
We acknowledged the financial support by DFG grants (KH 31/8-1, GR 1540/28-1, and IV 124/3-1) as well as the BMBF grant to REEBUS (WP6: Carbon fluxes in the water column, Grant Number 03F0815D). MI was funded by the HGF Young Investigator Group SeaPump “Seasonal and Regional Food Web Interactions with the Biological Pump”: VH-NG-1000. This work was further supported by the DFG-Research Center/Cluster of Excellence “The Ocean in the Earth System”: EXC-2077-390741603 (to NM and MI).
Conflict of Interest
The authors declare that the research was conducted in the absence of any commercial or financial relationships that could be construed as a potential conflict of interest.
Publisher’s Note
All claims expressed in this article are solely those of the authors and do not necessarily represent those of their affiliated organizations, or those of the publisher, the editors and the reviewers. Any product that may be evaluated in this article, or claim that may be made by its manufacturer, is not guaranteed or endorsed by the publisher.
Acknowledgments
The financial support provided by BMBF (03F0815D) to GF and MI through REEBUS WP-6 (MARE-N) as well as the supports of Bremen University, Jacobs University Bremen, Max Planck Institute for Marine Microbiology, IGB Berlin, Alfred Wegener Institute for Polar and Marine Research, Helmholtz 1223 Association, and the DFG-Research Center/Cluster of Excellence “The Ocean in the Earth System”: EXC-2077-390741603 are greatly acknowledged.
Footnotes
References
Alldredge, A. L., and Cohen, Y. (1987). Can microscale chemical patches persist in the sea? Microelectrode study of marine snow, fecal pellets. Science 235, 689–691. doi: 10.1126/science.235.4789.689
Azam, F., and Malfatti, F. (2007). Microbial structuring of marine ecosystems. Nat. Rev. Microbiol. 5, 782–791. doi: 10.1038/nrmicro1747
Baumgärtl, H., and Lübbers, D. W. (1973). “Platinum needle electrodes for polarographic measurement of oxygen and hydrogen,” in Oxygen Supply, eds M. Kessler et al. (Vienna: Urban and Schwarzenberg), 130–136.
Baumgärtl, H., and Lübbers, D. W. (1983). “Microcoaxial needle sensor for polarographic measurement of local O2 pressure in the cellular range of living tissue. Its construction and properties,” in Polarographic Oxygen Sensors, eds E. Gnaiger and H. Forstner (Berlin: Springer-Verlag), 37–65. doi: 10.1007/978-3-642-81863-9_4
Belcher, A., Iversen, M., Giering, S., Riou, S., Henson, S. A., Berline, L., et al. (2016). Depth-resolved particle-associated microbial respiration in the northeast Atlantic. Biogeosciences 13:4927. doi: 10.5194/bg-13-4927-2016
Berg, P., Risgaard-Petersen, N., and Rysgaard, S. (1998). Interpretation of measured concentration profiles in sediment pore water. Limnol. Oceanogr. 43, 1500–1510. doi: 10.4319/lo.1998.43.7.1500
Berg, P., Rysgaard, S., and Thamdrup, B. (2003). Dynamic modeling of early diagenesis and nutrient cycling. a case study in an artic marine sediment. Am. J. Sci. 303, 905–955. doi: 10.2475/ajs.303.10.905
Brand, A., Müller, B., Wüest, A., Dinkel, C., Revsbech, N. P., Nielsen, L. P., et al. (2007). Microsensor for in situ flow measurements in benthic boundary layers at submillimeter resolution with extremely slow flow. Limnol. Oceanogr. Methods 5, 185–191. doi: 10.4319/lom.2007.5.185
Bryant, L., McGinnis, D., Lorrai, C., Brand, A., Little, J., and Wüest, A. (2010). Evaluating oxygen fluxes using microprofiles from both sides of the sediment-water interface. Limnol. Oceanogr. Methods 8, 610–627. doi: 10.4319/lom.2010.8.0610
de Beer, D., van den Heuvel, J. C., and Ottengraf, S. P. P. (1993). Microelectrode measurements of the activity distribution in nitrifying bacterial aggregate. Appl. Environ. Microbiol. 59, 573–590. doi: 10.1128/aem.59.2.573-579.1993
Eichner, M. J., Klawonn, I., Wilson, S. T., Littmann, S., Whitehouse, M. J., Church, M. J., et al. (2017). Chemical microenvironments and single-cell carbon and nitrogen uptake in field-collected colonies of Trichodesmium under different pCO2. ISME J. 11, 1305–1317. doi: 10.1038/ismej.2017.15
Flemming, H. C., and Wuertz, S. (2019). Bacteria and archaea on Earth and their abundance in biofilms. Nat. Rev. Microbiol. 17, 247–260. doi: 10.1038/s41579-019-0158-9
Glud, R. N. (2008). Oxygen dynamics of marine sediments. Mar. Biol. Res. 4, 243–289. doi: 10.1080/17451000801888726
Glud, R. N., Gundersen, J. K., Jørgensen, B. B., Revsbech, N. P., and Schulz, H. D. (1994). Diffusive and total oxygen uptake of deep-sea sediments in the eastern South Atlantic Ocean: in situ and laboratory measurements. Deep Sea Res. 41, 1767–1788.
Gundersen, J., and Jørgensen, B. (1990). Microstructure of diffusive boundary layers and the oxygen uptake of the sea floor. Nature 345, 604–607. doi: 10.1038/345604a0
Hondzo, M., Feyaerts, T., Donovan, R., and O’Connor, B. L. (2005). Universal scaling of dissolved oxygen distribution at the sediment-water interface: a power law. Limnol. Oceanogr. 50, 1667–1676. doi: 10.4319/lo.2005.50.5.1667
Iversen, M. H., Nowald, N., Ploug, H., Jackson, G. A., and Fischer, G. (2010). High resolution profiles of vertical particulate organic matter export off Cape Blanc, Mauritania: degradation processes and ballasting effects. Deep Sea Res. 57, 771–784. doi: 10.1016/j.dsr.2010.03.007
Iversen, M. H., Pakhomov, E. A., Hunt, B. P. V., van der Jagt, H., Wolf-Gladrow, D., and Klaas, C. (2017). Sinkers or floaters? Contribution from salp fecal pellets to the export during a large bloom event in the Southern Ocean. Deep Sea Res. 138, 116–125. doi: 10.1016/j.dsr2.2016.12.004
Iversen, M. H., and Ploug, H. (2010). Ballast minerals and the sinking carbon flux in the ocean: carbon-specific respiration rates and sinking velocity of marine snow aggregates. Biogeosciences 7, 2613–2624. doi: 10.5194/bg-7-2613-2010
Jackson, G. A. (2012). Seascapes: the world of aquatic organisms as determined by their particulate natures. J. Exp. Biol. 215, 1017–1030. doi: 10.1242/jeb.059105
Jørgensen, B. B. (2000). “Bacteria and marine biogeochemistry,” in Marine Geochemistry, eds H. D. Schulz and M. Zabel (Berlin: Springer), 169–206. doi: 10.1007/3-540-32144-6_5
Jørgensen, B. B., and Revsbech, N. P. (1985). Diffusive boundary layers and the oxygen uptake of sediments and detritus. Limnol. Oceanogr. 1, 111–122. doi: 10.4319/lo.1985.30.1.0111
Kiørboe, T., Ploug, H., and Thygesen, U. H. (2001). Fluid motion and solute distribution around sinking aggregates. I. Small-scale fluxes and heterogeneity of nutrients in the pelagic environment. Mar. Ecol. Prog. Ser. 211, 1–13. doi: 10.3354/meps211001
Klawonn, I., Eichner, M. J., Wilson, S. T., Moradi, N., Thamdrup, B., Kümmel, S., et al. (2020). Distinct nitrogen cycling and steep chemical gradients in Trichodesmium colonies. ISME J. 14, 399–412. doi: 10.1038/s41396-019-0514-9
Klawonn, I., Bonaglia, S., Brüchert, V., and Ploug, H. (2015). Aerobic and anaerobic nitrogen transformation processes in N2-fixing cyanobacterial aggregates. ISME J. 9, 1456–1466. doi: 10.1038/ismej.2014.232
Kühl, M., and Revsbech, N. P. (2001). “Biogeochemical microsensors for boundary layer studies,” in The Benthic Boundary Layer: Transport Processes and Biogeochemistry, eds B. B. Boudreau and B. B. Jørgensen (Oxford: Oxford University Press), 180–210.
Lemburg, J., Wenzhöfer, F., Hofbauer, M., Färber, P., and Meyer, V. (2018). “Benthic crawler NOMAD – increasing payload by low-density design,” in Proceedings of the OCEANS 2018 MTS/IEEE, Kobe.
Lewandowski, Z. (2000). Notes on biofilm porosity. Water Res. 34, 2620–2624. doi: 10.1016/s0043-1354(00)00186-x
Moradi, N., Liu, B., Iversen, M., Kuypers, M. M., Ploug, H., and Khalili, A. (2018). A new mathematical model to explore microbial processes and their constraints in phytoplankton colonies and sinking marine aggregates. Sci. Adv. 4:eaat1991. doi: 10.1126/sciadv.aat1991
O’Connor, B. L., and Harvey, J. W. (2008). Scaling hyporheic exchange and its influence on biogeochemical reactions in aquatic ecosystems. Water Resour. Res. 44:W12423. doi: 10.1029/2008WR007160
Paerl, H. W., and Bebout, B. M. (1988). Direct measurement of O2-depleted microzones in marine oscillatoria: relation to N2 fixation. Science 241, 442–445. doi: 10.1126/science.241.4864.442
Ploug, H. (2001). Small-scale oxygen fluxes and remineralization in sinking aggregates. Limnol. Oceanogr. 46, 1624–1631. doi: 10.4319/lo.2001.46.7.1624
Ploug, H., and Grossart, H. P. (2000). Bacterial growth and grazing on diatom aggregates: respiratory carbon turnover as a function of aggregate size and sinking velocity. Limnol. Oceanogr. 45, 1467–1475. doi: 10.4319/lo.2000.45.7.1467
Ploug, H., Hietanen, S., and Kuparinen, J. (2002). Diffusion and advection within and around sinking, porous diatom aggregates. Limnol. Oceanogr. 47, 1129–1136. doi: 10.4319/lo.2002.47.4.1129
Ploug, H., and Jørgensen, B. B. (1999). A net-jet flow system for mass transfer and microsensor studies of sinking aggregates. Mar. Ecol. Prog. Ser. 176, 279–290. doi: 10.3354/meps176279
Ploug, H., Grossart, H. P., Azam, F., and Jørgensen, B. B. (1999). Photosynthesis, respiration, and carbon turnover in sinking marine snow from surface waters of Southern California Bight: implications for the carbon cycle in the ocean. MEPS 179, 1–11. doi: 10.3354/meps179001
Ploug, H., Kühl, M., Buchholz-Cleven, B., and Jørgensen, B. B. (1997). Anoxic aggregates – an ephemeral phenomenon in the pelagic environment? Aquat. Microb. Ecol. 13, 285–294. doi: 10.3354/ame013285
Reichert, P. (1994). AQUASIM: A tool for simulation and data analysis of aquatic systems. Water Sci. Tech. 30, 21–30. doi: 10.2166/WST.1994.0025
Reimers, C. E. (1987). An in situ microprofiling instrument for measuring interfacial pore water gradients: methods and oxygen profiles from the North Pacific Ocean. Deep-Sea Res. 34, 2019–2035.
Revsbech, N. P. (1983). “In Situ measurement of oxygen profiles of sediments by use of oxygen microelectrodes,” in Handbook on Polarographic Oxygen Sensors: Aquatic and Physiological Applications, eds E. Gnaiger and H. Forstner (Berlin: Springer-Verlag), 266–273.
Revsbech, N. P., and Jørgensen, B. B. (1986). “Microelectrodes: their use in microbial ecology,” in Advances in Microbial Ecology, ed. K. C. Marshall (New York, NY: Springer US), 293–352. doi: 10.1007/978-1-4757-0611-6_7
Revsbech, N. P., and Ward, D. M. (1983). Oxygen microelectrode that is insensitive to medium chemical composition: useinan acid microbial mat dominated by Cyanidium caldarium. Appl. Environ. Microbiol. 43, 755–759. doi: 10.1128/aem.45.3.755-759.1983
Røy, H., Huettel, M., and Jørgensen, B. B. (2002). The role of small-scale sediment topography for oxygen flux across the diffusive boundary layer. Limnol. Oceanogr. 47, 837–847. doi: 10.4319/lo.2002.47.3.0837
Røy, H., Huettel, M., and Jørgensen, B. B. (2004). Transmission of oxygen concentration fluctuations through the diffusive boundary layer overlaying aquatic sediment. Limnol. Oceanogr. 49, 686–692. doi: 10.4319/lo.2004.49.3.0686
Seymour, J. R., Amin, S. A., Raina, J.-B., and Stocker, R. (2017). Zooming in on the phycosphere: the ecological interface for phytoplankton–bacteria relationships. Nat. Microbiol. 2:17065.
Shanks, A. L., and Edmondson, E. W. (1989). Laboratory-made artificial marine snow: a biological model of the real thing. Mar. Biol. 101, 463–470. doi: 10.1007/bf00541648
Simon, M., Grossart, H. P., Schweitzer, B., and Ploug, H. (2002). Microbial ecology of organic aggregates in aquatic ecosystems. Aquat. Microb. Ecol. 28, 175–211. doi: 10.3354/ame028175
Stief, P., Kamp, A., Thamdrup, B., and Glud, R. N. (2016). Anaerobic nitrogen turnover by sinking diatom aggregates at varying ambient oxygen levels. Front. Microbiol. 7:98. doi: 10.3389/fmicb.2016.00098
Urban, N. R., Dinkel, C., and Wehrli, B. (1997). Solute transfer across the sediment surface of a eutrophic lake: I. Porewater profiles from dialysis samplers. Aquat. Sci. 59, 1–25. doi: 10.1007/BF02522546
Wenzhöfer, F., and Glud, R. N. (2002). Benthic carbon mineralization in the Atlantic: a synthesis based on in situ data from the last decade. Deep Sea Res. 49, 1255–1279.
Wenzhöfer, F., Holby, O., and Kohls, O. (2001). Deep penetrating benthic oxygen profiles measured in situ by oxygen optodes. Deep Sea Res. I 48, 1741–1755. doi: 10.1016/s0967-0637(00)00108-4
Wenzhöfer, F., Kazumasa, O., Middelboe, M., Turnewitsch, R., Toyofuku, T., Kitazato, H., et al. (2016). Benthic carbon mineralization in hadal trenches: assessment by in situ O2 microprofile measurements. Deep Sea Res. I 116, 276–286. doi: 10.1016/j.dsr.2016.08.013
Keywords: diffusive flux, computational model, microsensor profiling, deep-sea sediment, marine aggregates, interface position, microbial O2 respiration
Citation: Moradi N, Klawonn I, Iversen MH, Wenzhöfer F, Grossart H-P, Ploug H, Fischer G and Khalili A (2021) A Novel Measurement-Based Model for Calculating Diffusive Fluxes Across Substrate-Water Interfaces of Marine Aggregates, Sediments and Biofilms. Front. Mar. Sci. 8:689977. doi: 10.3389/fmars.2021.689977
Received: 01 April 2021; Accepted: 05 July 2021;
Published: 18 August 2021.
Edited by:
Antonio Cobelo-Garcia, Consejo Superior de Investigaciones Científicas (CSIC), SpainReviewed by:
Miguel Caetano, Portuguese Institute for Sea and Atmosphere (IPMA), PortugalJeffrey Cornwell, University of Maryland Center for Environmental Science (UMCES), United States
Copyright © 2021 Moradi, Klawonn, Iversen, Wenzhöfer, Grossart, Ploug, Fischer and Khalili. This is an open-access article distributed under the terms of the Creative Commons Attribution License (CC BY). The use, distribution or reproduction in other forums is permitted, provided the original author(s) and the copyright owner(s) are credited and that the original publication in this journal is cited, in accordance with accepted academic practice. No use, distribution or reproduction is permitted which does not comply with these terms.
*Correspondence: Nasrollah Moradi, nmoradi@marum.de
†Present address: Isabell Klawonn, Leibniz Institute for Baltic Sea Research (IOW), Rostock, Germany